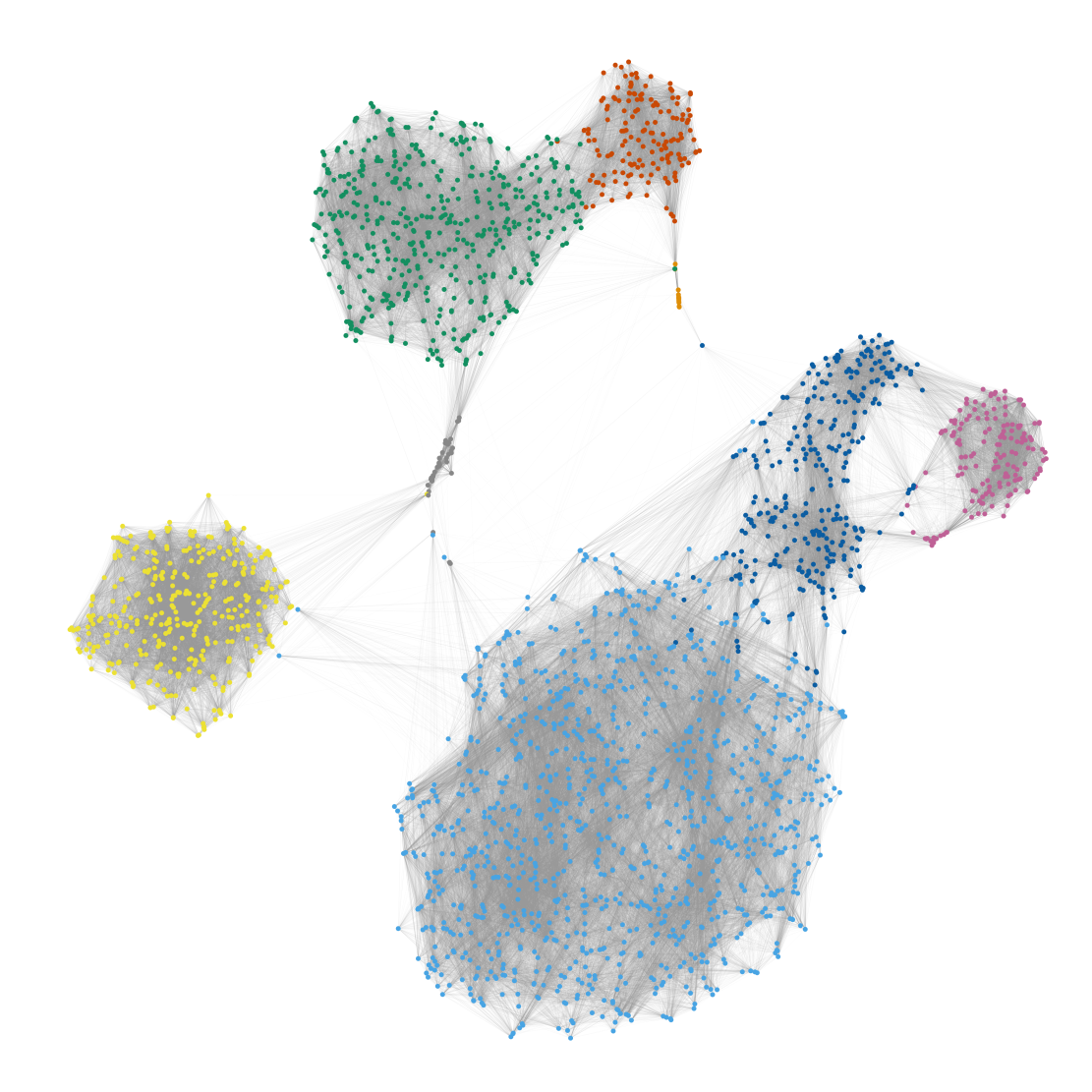
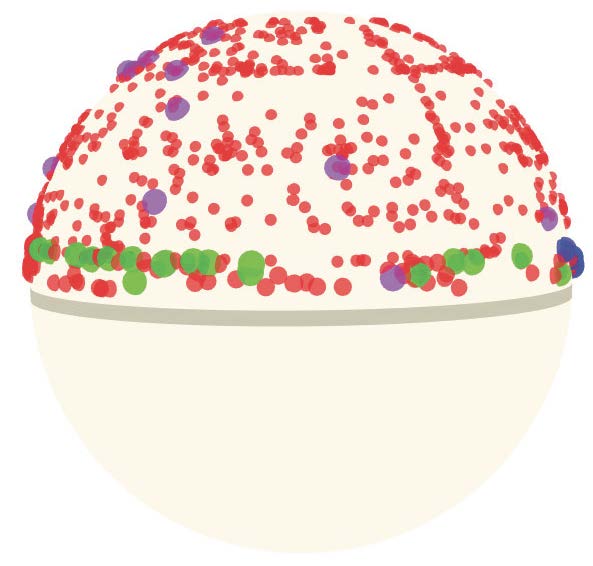
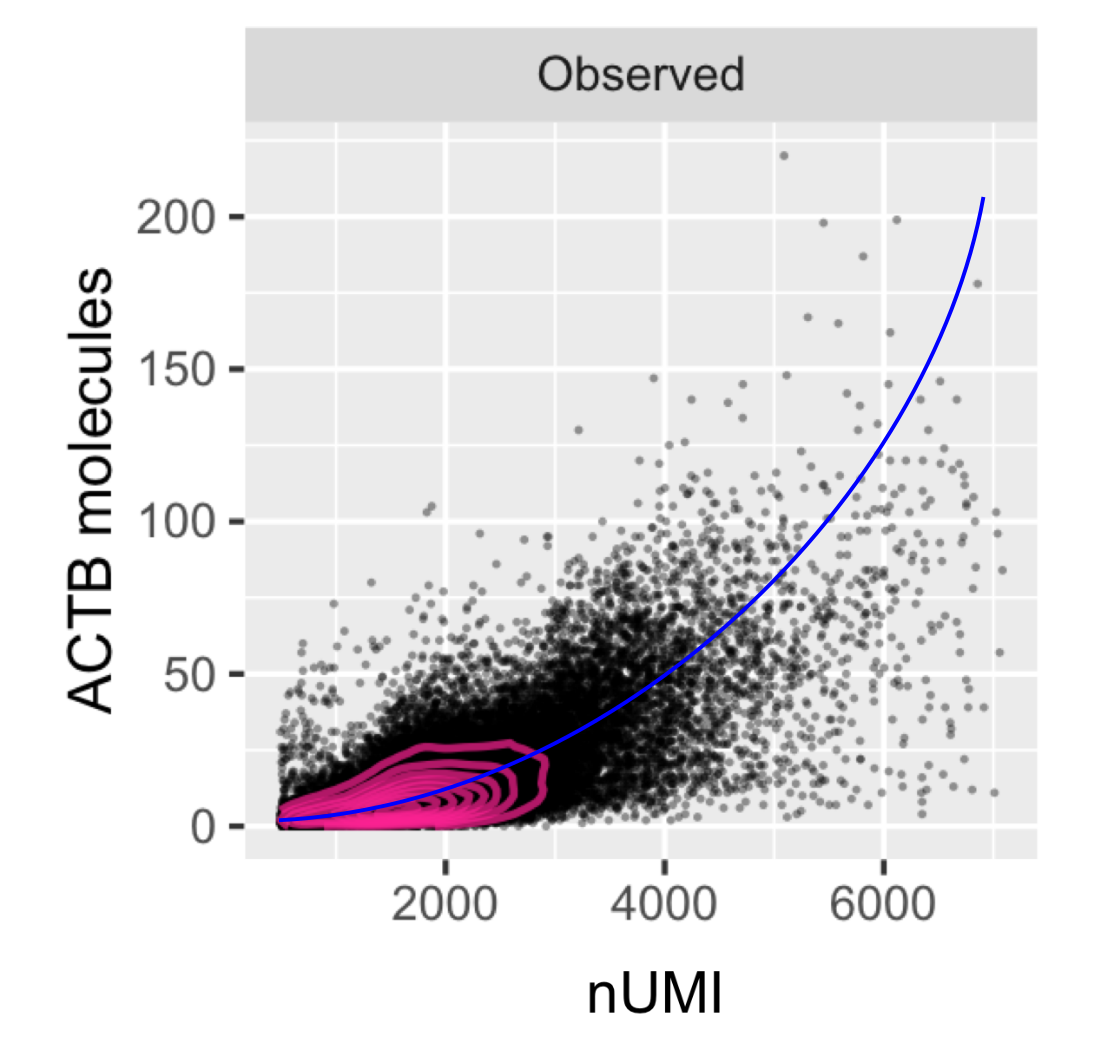
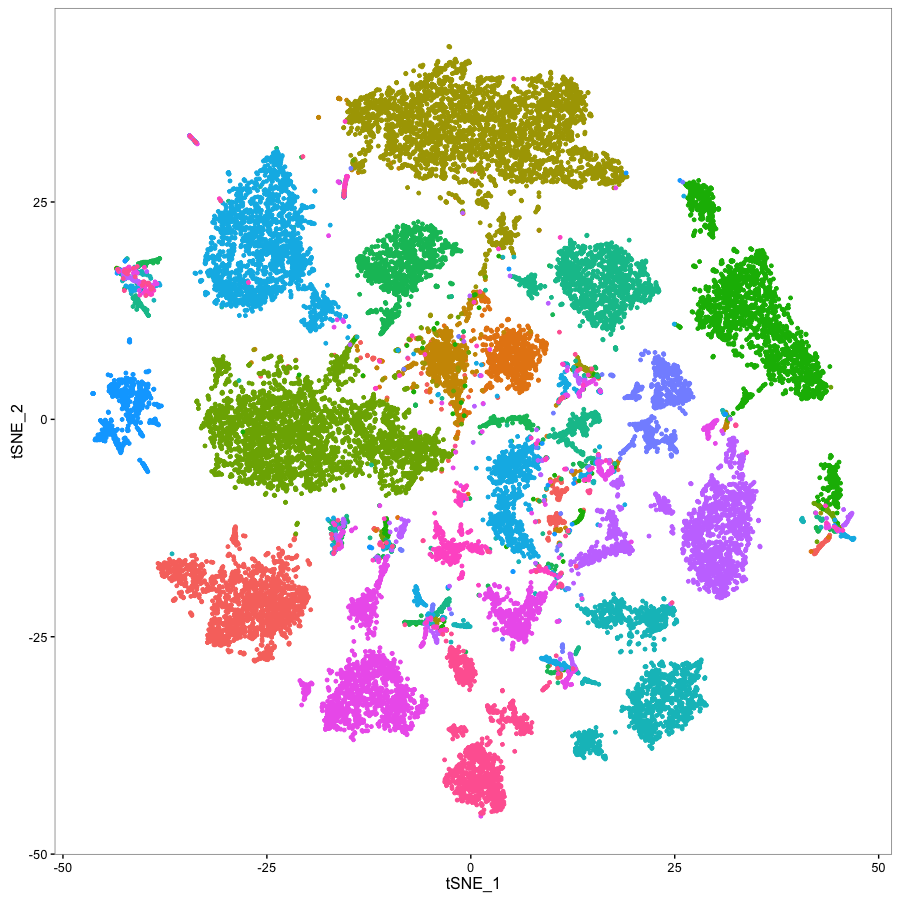
Statistical methods for single cell genomics
Recent advances in molecular biology and microfluidics have enabled us to individually sequence the molecular contents of thousands of single cells. These datasets promise to transform our understanding of cellular diversity, but they are incomplete. Firstly, they contain information for only a small fraction of molecules in a cell - necessitating new methods capable of handling extensive technical noise. Secondly, they contain only molecular information about the cell, and lack crucial metadata about a cell’s environment, lineage, and interactions, that are essential towards understanding and predicting its behavior. To address these challenges, we are applying powerful tools in statistical inference and machine-learning to single cell data. We have recently developed a set of computational methods that can integrate information across multiple data types, modalities, and experimental conditions (Stuart*, Butler* et al., Cell 2019, Butler et al., Nat Biotech. 2018.
Moving forward, we have a particular interest in new technologies that enable single-cell measurements extending beyond the transcriptome, including protein, chromatin, and functional assays. For example, in collaboration with the Technology Innovation Lab at NYGC, we recently introduced CITE-seq (Stoeckius et al., Nat. Methods 2017), a new technology to simultaneously measure the transcriptome alongside hundreds of surface proteins in single-cells. We are actively developing new experimental strategies to profile additional modalities, alongside analytical approaches to define cellular identity based on multiple sources of information. We hope that these tools will be valuable not only across multiple projects in the lab, but also to the broader community.
Integrated analysis of cellular decision-making
Cellular diversity increases dramatically during differentiation, as a single progenitor can give rise to a breathtaking diversity of cell types. How do progenitor cells choose their terminal fates? Through single cell analysis, we are exploring the intrinsic and extrinsic factors which drive cellular decision-making. Single cell RNA-seq enables powerful approaches to reconstruct developmental trajectories, but we are also building an integrated framework to understand how a cell’s spatial localization, epigenomic landscape, and parental lineage influence its behavior and fate. Through projects driven both within the lab, and collaboratively across NYC, we focus on the development of the mammalian immune and nervous systems. For example, in collaboration with Dr. Gord Fishell, we recently described the initial molecular steps taken by embryonic interneuron progenitors as they commit to their adult fates (Mayer*, Hafemeister*, Bandler* et al., Nature 2018).
Deconvolution of autoimmune disease and hematalogical malignancies
The immune system strikes a delicate balance between immunity and tolerance, enabled by diverse subsets of interdependent and intercommunicating cells. Abnormal immune responses lead to autoimmune disease, and this extensive cellular heterogeneity can obscure the subpopulations and molecular pathways that drive disease progression and treatment. Working with immunologists and clinical collaborators at NYU, we have established pipelines for the generation of multi-omic, single-cell, and spatially-resolved datasets, aiming to identify and characterize pathogenic populations. We have a particular interest in understanding how interactions between cells, driven by spatial and environmental influences, influence disease phenotypes. For example, in collaboration with the Hospital for Special Surgery, we recently identified a spatially restricted population of fibroblasts in synovial tissue samples from rheumatoid arthritis patients (Stephenson*, Donlin*, Butler* et al., Nat. Communications 2018). More generally, we hope to build new experimental and computational tools that will advance and democratize clinical applications for single cell genomics.
Understanding the spatial structure and organization of the human body
We lead a ‘Mapping Center’ as part of the NIH Human Biomolecular Atlas Program (HuBMAP), which aims to develop an open and global platform to map healthy cells in the human body. With our collaborators John Marioni and Aviv Regev, we are building a ‘common coordinate framework’, an underlying map of of tissues and organs. The CCF will enable robust comparisons of spatially-resolved differences across individuals, even in the presence of extensive anatomical variation. As described in our recent review article (Rood et al., Cell 2019), we hope that this reference framework will lead to a deeper understanding of the structural organization of the human body in both health and disease.