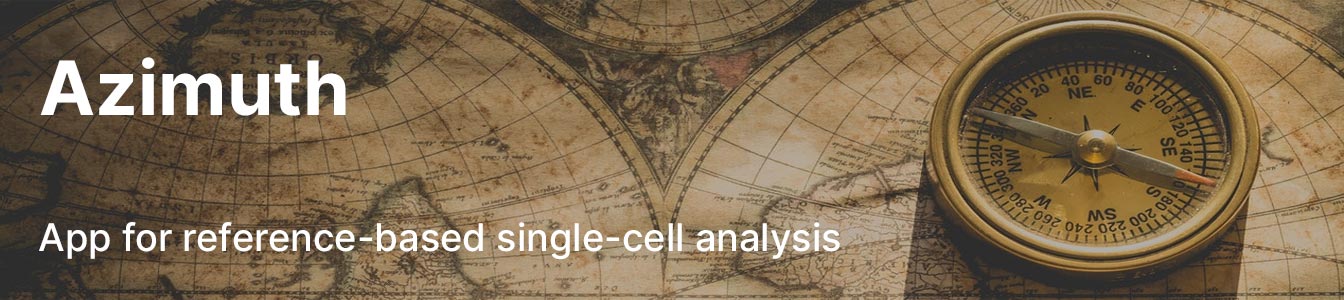
Azimuth is a web application that uses an annotated reference dataset to automate the processing, analysis, and interpretation of a new single-cell RNA-seq experiment. Azimuth leverages a ‘reference-based mapping’ pipeline that inputs a counts matrix of gene expression in single cells, and performs normalization, visualization, cell annotation, and differential expression (biomarker discovery). All results can be explored within the app, and easily downloaded for additional downstream analysis.
Since our initial release Azimuth has been updated and released as part of the Human Biomolecular Atlas Program, and is accessible at: azimuth.hubmapconsortium.org
If you have previously uploaded your data to - we recommend re-mapping your data with the latest version at azimuth.hubmapconsortium.org. The updated version includes the following new features:
- Reference datasets are available for four organs
- Improved mapping results, particularly when the query dataset represents a subset of the reference diversity (i.e. mapping sorted T cells onto the PBMC reference)
- Interactive plots and visualizations
- Ability to annotate cells at multiple levels of resolution/granularity
- New QC metrics (replacing the previous pseudobulk correlation test)